AI in Fintech: Benefits, Key Applications and Future Trends
Artificial Intelligence (AI) and Machine Learning (ML) are revolutionizing the fintech industry, changing how we manage finances, interact with financial services, and ensure security. With the rapid digital transformation in finance, AI applications in fraud detection, customer support, and personalized financial planning are not just helpful—they are becoming essential. This blog explores the evolving role of AI and ML in fintech, the benefits they bring, popular applications, and the trends that will shape the future of the industry.
Introduction to AI in Fintech
AI in fintech has advanced from simple automation to complex systems that predict, analyze, and prevent risks in real time. AI-powered fraud detection, for instance, protects users and financial institutions from financial crimes by analyzing historical data to flag unusual patterns and behaviors. This is particularly crucial as digital transactions grow and cyber threats evolve.
Additionally, AI transforms risk assessment in fintech. With machine learning, banks and fintech companies can analyze vast data sets, improving lending and investment decisions. This data-driven approach reduces exposure to risky assets and enhances credit scoring models, broadening financial access for underserved communities. As AI continues advancing, its applications in financial services will grow, creating more secure and efficient solutions.
Benefits of AI in Financial Services and Operations
AI offers fintech companies multiple benefits, from enhancing security to streamlining operations. Let’s look at some of the key advantages in more detail:
- AI-Powered Fraud Detection: Securing Fintech Transactions
Fraud detection is one of AI’s most critical applications in financial services. Unlike traditional rule-based systems, AI-driven models continuously adapt, learning from each transaction to improve accuracy. This adaptability is key to staying ahead of cybercriminals. As fraud tactics evolve, AI’s adaptability ensures fintech companies stay one step ahead, safeguarding both their assets and their customers’ information. - Optimized Decision-Making and Risk Management
The ability of AI to process large volumes of data has transformed decision-making across finance. Investment firms, for instance, use machine learning to identify trends and predict market changes, enhancing returns. Smaller companies can also leverage AI to make data-driven decisions, making them more competitive. AI models further refine credit assessments by analyzing variables beyond traditional scores, such as transaction history, providing a more comprehensive view of creditworthiness. - Operational Efficiency and Cost Savings
Automating routine tasks—such as data entry, transaction monitoring, and reporting—can dramatically reduce operational costs for fintech companies. AI-powered automation decreases human error, accelerates processing times, and frees employees to focus on high-value tasks. For example, in customer service, chatbots powered by natural language processing (NLP) can handle common inquiries and basic requests 24/7, significantly reducing the workload on human agents. Not only does this increase operational efficiency, but it also provides a faster, more consistent customer experience. - Improved Customer Personalization
Personalization is a growing demand in fintech, and AI is the technology making it possible. By analyzing individual user behavior, preferences, and financial goals, AI can tailor financial advice, product recommendations, and even account settings to each customer’s needs. This personalized approach not only improves customer satisfaction but also increases user engagement and retention by making financial services more relevant and accessible.
AI Applications in Fintech : Real-world Case Studies
AI applications in fintech are transforming the financial industry with cutting-edge solutions. Let’s examine the five primary AI applications that are revolutionizing fintech, how they work, and their impact with real-world case studies.
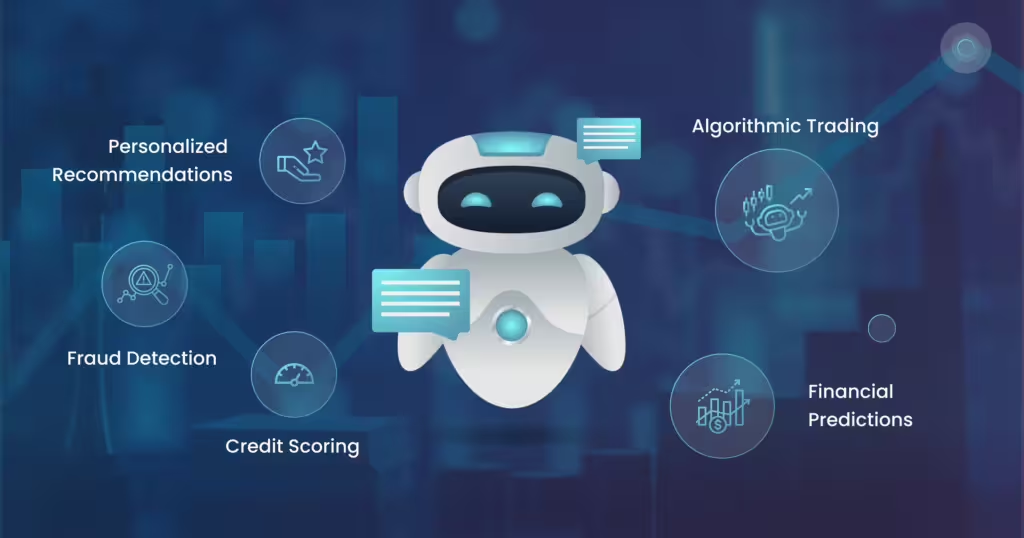
1. Fraud Detection: Real-Time Adaptation to Evolving Threats
Fraud detection remains one of the most crucial applications of AI in fintech. Unlike traditional systems, which rely on static rule-based frameworks, AI-driven fraud detection systems utilize machine learning algorithms that analyze vast datasets to detect anomalies and suspicious behavior in real-time. These systems continuously learn and adapt, enabling them to detect evolving fraud patterns more accurately.
Example: PayPal, a leader in digital payments, uses AI to monitor millions of transactions in real-time, identifying unusual patterns that might indicate fraud. PayPal’s system leverages machine learning models that can adapt to new types of fraud and learn from previous cases. This AI-powered approach has reduced its fraud rate to 0.32%, one of the lowest in the industry.
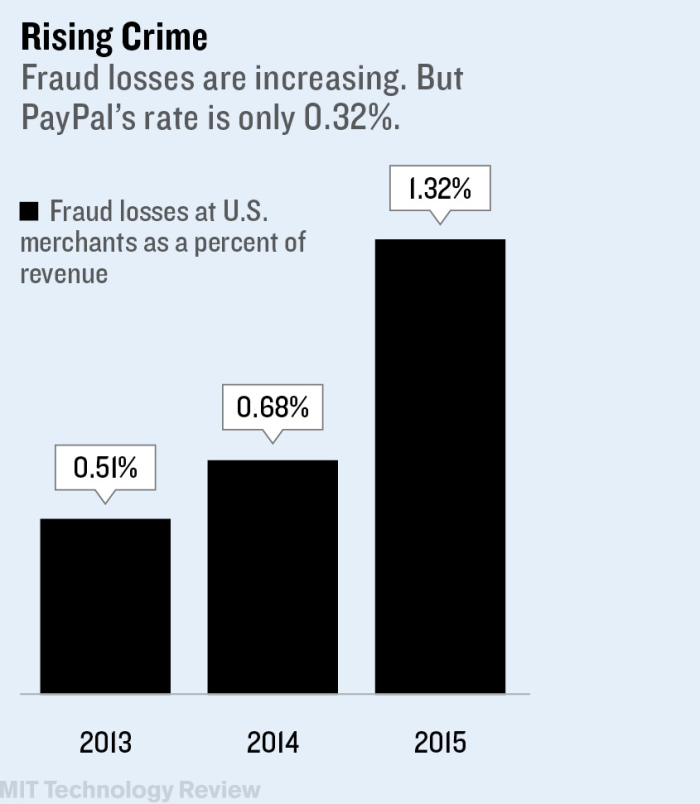
Benefits: AI-based fraud detection minimizes financial and reputational losses by proactively identifying fraudulent activities. It also improves customer trust, as users feel their transactions are safeguarded by advanced, intelligent systems.
2. Customer Personalization: Tailoring Financial Services to Individual Needs
Customer personalization is a game-changer in fintech, and AI is at the forefront of making this possible. By analyzing customer behavior, spending patterns, and personal preferences, AI algorithms create highly tailored financial services. Whether it’s personalized savings advice, investment recommendations, or customized loan products, AI helps financial institutions provide unique experiences for each customer.
Example: Wealthfront, a popular robo-advisor, uses AI to create customized investment portfolios that align with each user’s risk tolerance, financial goals, and market trends. Wealthfront’s personalization has been a major factor in its growth, managing over $25 billion in assets and achieving a 33% higher retention rate than traditional advisory services.
Benefits: AI-powered personalization enhances customer engagement and satisfaction by making financial services more relevant to individual needs. It also strengthens loyalty, as users are more likely to stay with a service that feels uniquely suited to their preferences and financial goals.
3. Algorithmic Trading: Leveraging High-Frequency Data for Real-Time Decisions
Algorithmic trading, especially high-frequency trading (HFT), is another prominent AI application that revolutionizes how financial markets operate. In HFT, AI algorithms analyze massive volumes of market data in real-time to make trading decisions within milliseconds. These models identify patterns in price movements, volatility, and trading volumes, allowing them to execute trades at the optimal moment to maximize returns and minimize risk.
Example: Citadel Securities, a major player in quantitative trading, uses AI to manage over 25% of U.S. equities trading volume daily. Its AI-driven HFT system processes data at lightning speed, enabling trade execution in under one millisecond. This rapid decision-making capability has contributed to Citadel’s impressive returns and a strong reputation in the industry.
Benefits: AI in algorithmic trading reduces human error, enhances trading speed, and optimizes profitability by reacting instantly to market changes. This application is especially valuable in volatile markets, where delays can lead to substantial financial losses.
4. Regulatory Compliance (RegTech): AI for Real-Time Compliance Monitoring
Regulatory compliance is a complex, ever-evolving challenge in the financial industry, with global institutions needing to adhere to numerous regional regulations. AI-powered Regulatory Technology (RegTech) automates compliance tasks like Anti-Money Laundering (AML) checks, Know Your Customer (KYC) verification, and transaction monitoring. By analyzing vast datasets, AI-driven RegTech solutions can identify potential non-compliance issues and notify institutions in real-time, enabling a proactive approach to regulatory adherence.
Example: HSBC, one of the world’s largest banks, adopted AI-based RegTech solutions to enhance its AML processes. Using machine learning, HSBC’s system monitors transactions and analyzes patterns to identify potential money laundering activities. This has reduced the time HSBC spends on transaction monitoring by 20%, improving both efficiency and accuracy.
Benefits: AI-driven compliance solutions save financial institutions time and reduce the risk of regulatory fines. By enhancing transparency and data security, RegTech strengthens customer trust and supports financial institutions in maintaining high ethical standards.
5. Risk Assessment and Underwriting: Expanding Financial Access with AI
Risk assessment and underwriting have traditionally relied on conventional credit scores, which can exclude individuals without a credit history. AI transforms this process by using alternative data—such as income patterns, transaction behaviors, and even social data—to evaluate creditworthiness. This more nuanced approach enables lenders to reach underserved communities and provide financial access to a broader range of customers.
Example: Tala, a fintech company focused on underserved markets, uses AI to assess credit risk by analyzing non-traditional data, such as mobile usage and social behavior. Tala’s AI models enable the company to offer microloans to over six million customers in emerging markets like Kenya and the Philippines, achieving a repayment rate of over 90%.
Benefits: AI-driven underwriting expands financial inclusion by providing access to credit for individuals who might not qualify under traditional scoring models. This approach is transformative for underserved populations and empowers fintech companies to tap into new markets, driving growth and social impact.
AI Trends Shaping the Future of Fintech
As AI technology evolves, several emerging trends are set to reshape the fintech landscape. Let’s explore some of these forward-looking trends in more depth:
- Predictive Analytics for Personalized Financial Services
Predictive analytics is one of the most promising areas of AI for fintech. By analyzing vast datasets, predictive models can forecast user behaviors, helping institutions anticipate customer needs and preferences. For instance, AI can detect patterns that indicate when a customer may need financial assistance or is likely to invest in a new product. Such predictive capabilities allow fintech companies to offer proactive solutions, making financial planning a more engaging and supportive experience for users. This trend toward predictive personalization is likely to deepen, as companies leverage increasingly sophisticated models to meet customer expectations.
- Integration with Decentralized Finance (DeFi) and Blockchain
AI and blockchain technology are converging, particularly in the field of decentralized finance (DeFi). DeFi platforms leverage blockchain’s transparency and decentralization, while AI optimizes processes like transaction validation, fraud detection, and market analysis. For example, AI-powered smart contracts can execute financial agreements based on real-time data, ensuring faster, more reliable transactions. The integration of AI in DeFi has the potential to create financial ecosystems that are not only secure but also highly efficient, opening up new opportunities for customers who prefer a decentralized approach to finance.
- Hybrid AI Models for Enhanced Decision-Making
The next generation of AI models will combine various learning techniques, such as supervised, unsupervised, and reinforcement learning, to improve decision-making accuracy. In fintech, hybrid models can analyze diverse data sources, providing more reliable risk assessments, market predictions, and customer insights. By incorporating hybrid AI, fintech companies can enhance the effectiveness of their services, offering more robust, real-time solutions that adapt to a constantly changing financial landscape.
- AI for Financial Inclusion
One of the most exciting potentials of AI in fintech is its ability to foster financial inclusion. AI can analyze non-traditional data to provide financial services to those who lack access to traditional banking systems. By examining alternative data points, such as mobile phone usage or spending behavior, AI models can offer credit to underserved populations, helping to bridge the gap in financial accessibility. This trend is particularly impactful in developing markets, where traditional banking infrastructure may be limited but mobile technology is widespread.
- Robo-Advisors: The Future of Investment Management
Robo-advisors are set to revolutionize investment management, making financial planning and investing more accessible, efficient, and tailored to individual needs. These AI-powered platforms provide automated, algorithm-driven financial planning services with minimal human intervention. By analyzing a client’s financial goals, risk tolerance, and market conditions, robo-advisors can build, monitor, and rebalance investment portfolios in real-time.
Conclusion
The transformative power of AI in fintech is evident across applications that enhance fraud detection, streamline regulatory compliance, personalize customer experiences, and bring advanced investment management to everyone. As fintech companies adapt to the demands of a digital-first world, AI technologies will remain a core driver of security, efficiency, and customer satisfaction, shaping the future of financial services.
Ready to take your fintech solutions to the next level with AI? QSoft specializes in cutting-edge AI and machine learning solutions tailored to the unique needs of financial services. Contact us to explore how we can help integrate AI into your operations—from fraud detection and compliance to predictive analytics and personalized customer experiences.